Skills Intelligence
Skills intelligence is about turning this chaos into clarity. But what is it really? What does it take to implement? And why do so many organizations get it wrong?
What Is Skills Intelligence?
Let’s define it. Skills intelligence is the process of collecting, analyzing, and applying data about the skills your workforce currently has, the skills they’re developing, and the skills your organization actually needs — now and in the future.
It includes three major components:
- Skills Discovery: Mapping out what skills exist in your workforce today.
- Skills Gap Analysis: Understanding what skills are missing, declining, or becoming obsolete.
- Skills Forecasting: Anticipating what capabilities will be critical as the business evolves.
Effective strategies for leveraging skills intelligence are crucial for workforce development and talent management, including targeted approaches for training, hiring, and performance improvement.
Done well, it lets leaders answer tough questions with hard data, not gut feelings:
- Do we have enough AI-literate talent to deliver on our product roadmap?
- Who can step into leadership if someone leaves next quarter?
- Where are we vulnerable if our top tech leads walk out?
Why Traditional Talent Models Don’t Cut It
Companies have traditionally built corporate structures for job roles, not skill agility. Most HR systems still cling to job architectures from the last decade — hierarchical, rigid, and dangerously optimistic about how clearly roles map to actual work.
Let’s look at some of the outdated assumptions:
1. “Job titles = capabilities”
Wrong. Titles tell you almost nothing about what a person can actually do. A “Product Owner” in one company could be a strategic lead; in another, they’re writing Jira tickets and doing QA. Job titles often fail to reflect the true capabilities required for a particular role.
2. “Resumes = reality”
Resumés are aspirational documents. People oversell or undersell themselves all the time. Recruiting efforts can be hindered by these inaccuracies, as resumés do not always accurately reflect the skills of candidates. Your internal systems need to ground those claims in real performance data.
3. “Degrees = skills”
Dangerously false. Just because someone has a Master’s in Data Science doesn’t mean they can build production-ready ML models. Degrees often fail to accurately reflect the skill sets that employees possess. A self-taught engineer with a portfolio of working tools is often more valuable than someone with a paper credential.
This is where skills intelligence flips the model. Instead of assuming job roles define capability, it starts from what people can actually do — mapped across projects, outputs, learning activity, peer endorsements, and real-world application.
What Skills Intelligence Looks Like in Practice
Let’s drop the theory and get practical. Here’s how organizations with real skills intelligence systems operate:
1. Live, Dynamic Skills Maps
Skills are tagged, categorized, and updated continuously to reflect the latest trends — not once a year during performance reviews. Employees can self-report skills, but those inputs are validated through peer reviews, manager ratings, training outcomes, and observed behavior on projects.
Example: A software engineer lists “Python” as a skill. The system knows they’ve completed three complex back-end projects in Flask and Django, received positive feedback from two team leads, and contributed to an open-source tool. That’s not just a tag — that’s verified intelligence.
2. Skills Graphs, Not Spreadsheets
Forget static org charts. The most advanced systems use skills graphs — dynamic, visual maps showing how skills relate across roles, teams, and business units. These graphs let you find hidden experts, potential mentors, or unexpected candidates for a new initiative by gathering data from multiple sources and various platforms.
3. Real-Time Gap Analysis
Skills intelligence surface not only what you have but also what you don’t. If you’re building a new data product, and your team lacks production-level ML operations expertise, the system tells you immediately and offers internal mobility options, contractors, or learning paths. This enables you to make informed decisions based on accurate skills data, optimizing your talent management strategies.
4. Workforce Planning Based on Reality
Instead of vague workforce plans, leadership can forecast future needs tied directly to strategy. Launching in Latin America? You’ll need local legal expertise, Spanish fluency, and cross-cultural marketing skills. The system tells you how many people in the company have them, and how quickly others could be upskilled to align with common goals.
How to Build a Skills Intelligence System
It’s easy to get this wrong. Many organizations throw AI at the problem, buy expensive “talent cloud” software, or outsource to consulting firms who deliver 200-slide decks and zero usable insight.
To truly succeed, it’s crucial to identify and cultivate employees’ skills through tailored training programs that provide valuable learning opportunities.
Here’s how to do it right:
1. Start with a Clear Skills Taxonomy
You can’t manage what you don’t define. Start by creating a flexible, evolving taxonomy of skills relevant to your business. Avoid generic libraries — tailor it to your actual workflows and tech stack. And accept that this is a living structure. New skills will emerge; old ones will disappear. Develop this taxonomy continually to ensure it adapts to the changing needs of your organization.
2. Combine Multiple Data Sources
Don’t rely on self-assessments alone. Blend data from:
- Learning platforms (e.g., course completions, assessments)
- Project management tools (e.g., tasks completed, code pushed)
- Performance reviews and 360 feedback
- Manager evaluations
- External certifications
3. Involve Employees in the Process
skills mapping a two-way process: employees get visibility into how their skills are used and grown, and they help validate or challenge the data collected. Transparency builds trust — and better data quality. Additionally, this approach can assist employees by identifying knowledge gaps and providing personalized resources to help them perform unfamiliar tasks.
4. Tie Skills Intelligence to Real Outcomes
Don’t let this become another HR report. Tie your skills data to tangible business outcomes:
- Recruitment: Enhance talent acquisition by matching internal talent to open roles before posting externally.
- L&D: Fund learning programs that actually close business-critical gaps.
- Succession planning: Identify future leaders based on demonstrated capabilities.
- Innovation: Form agile project teams based on skill clusters, not org charts.
5. Avoid Vanity Metrics
A skills dashboard is not a solution. It’s a mirror. Don’t just track “most common skills” or “courses completed.” Look at deployment: how often are skills used in real work? How many are critical to revenue or compliance? Where are you exposed if people leave? Understand the value of tracking the deployment of skills to optimize workforce management and improve operational outcomes.
Risks and Blind Spots
Let’s not pretend this is a perfect science. Being aware of biases and privacy concerns is crucial, as skills intelligence have real limitations.
- Bias in Data Collection: If your data sources are biased (e.g., managers favoring certain groups, training access skewed by location), your skills model will reflect and reinforce those biases. This can lead to inaccurate skills data, which in turn fails to identify and address knowledge gaps effectively.
- Privacy and Consent: Collecting granular skills data feels invasive if mishandled. Be transparent about what’s collected, why, and how it’s used. Always give employees control over their profiles and be clear about how documenting employee skills can benefit their career development.
- Over-Reliance on AI:Artificial intelligence can help classify and analyze skill data, but it shouldn’t make talent decisions on its own. Human judgment still matters, especially in ambiguous areas like leadership potential or creative problem-solving.
- Obsolescence Risk: Skills data has a short half-life. A person’s capabilities change faster than your system updates. That’s why the model needs constant refreshment and developed strategies to keep the skills data current.
Conclusion
Skills intelligence isn’t a magic tool — it’s a lens. A way of seeing your workforce more clearly, managing it more responsibly, and preparing it for a future that doesn’t sit still. Organizations that get it right don’t just “optimize talent” — they build a workforce that’s adaptable, empowered, and aligned with actual business needs. They stop hiring for yesterday’s problems and start building capabilities for tomorrow’s opportunities. But it takes more than dashboards. It requires a shift in thinking: from jobs to skills, from structures to ecosystems, and from assumptions to evidence.
In a world where talent is the only true differentiator left, skills intelligence isn’t optional. It’s existential. Implementing skills intelligence leads to success by improving organizational performance and achieving desired outcomes through effective task prioritization and skills management.
Simplify the way people work and learn at the frontline
See the industry-leading how-to platform in a 30-minute live demo.
Learn more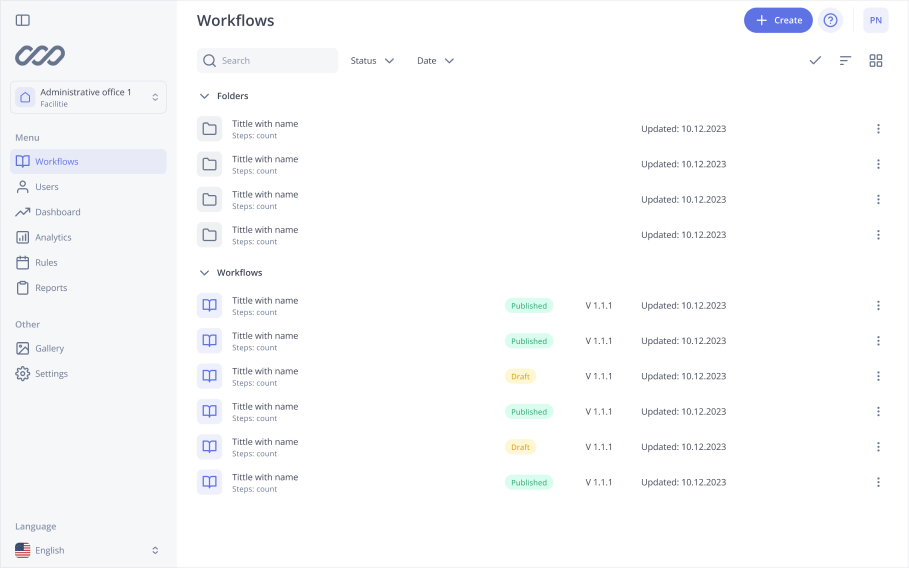
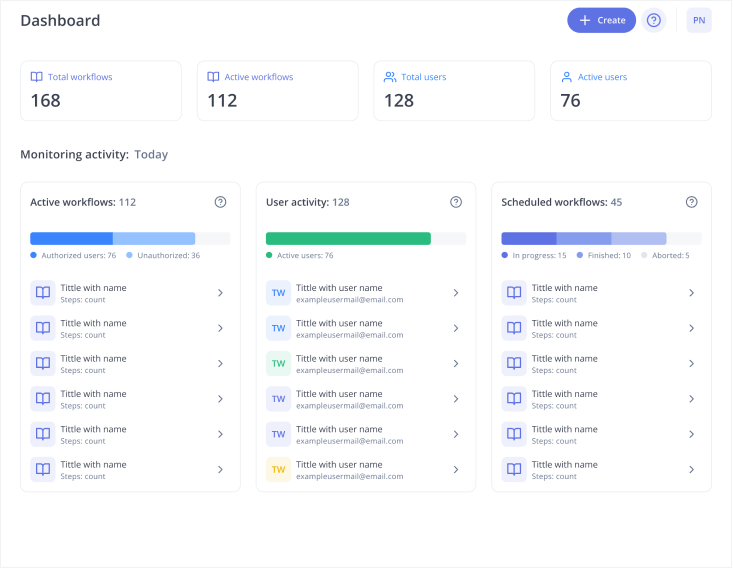